In recent years, there has been substantial interest in using deep neural networks (NNs) to improve the modeling and prediction of complex, multiscale, nonlinear dynamical systems such as turbulent flows and Earth’s climate. In idealized settings, there has been some progress for a wide range of applications from data-driven spatio-temporal forecasting to long-term emulation to subgrid-scale modeling.
However, to make these approaches practical and operational, i.e., scalable to real-world problems, a number of major questions and challenges need to be addressed. These include 1) instabilities and the emergence of unphysical behavior, e.g., due to how errors amplify through NNs, 2) learning in the small-data regime, 3) interpretability based on physics, and 4) out-of-distribution generalization (e.g., extrapolation to different parameters, forcings, and regimes) which is essential for applications to non-stationary systems such as a changing climate.
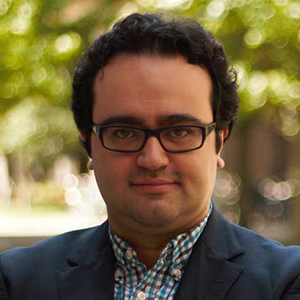
While some progress has been made in addressing (1)-(4), e.g., doing transfer learning for generalization, these approaches have been often ad-hoc, as currently there is no rigorous framework to analyze deep NNs and develop systematic and general solutions to (1)-(4).
In this talk, I will discuss some of the approaches to address (1)-(4), for example, once we identify spectral bias as the cause of instabilities in state-of-the-art weather models like Pangu-weather, GraphCast, and FourCastNet. Then I will introduce a new framework that combines the spectral (Fourier) analyses of NNs and nonlinear physics, and leverages recent advances in theory and applications of deep learning, to move toward rigorous analysis of deep NNs for applications involving dynamical systems. For example, this approach can guide and explain transfer learning and pruning in such applications. I will use examples from turbulence modeling and weather/climate prediction to discuss these methods and ideas.
Prof. Pedram Hassanzadeh is an associate professor in the Department of Geophysical Sciences and Committee on Computational and Applied Math at the University of Chicago. He received his MA and Ph.D. from University of California Berkeley. He was a Ziff Environmental Fellow at Harvard University before joining Rice University in 2016. He joined the University of Chicago in 2024. His research is at the intersection of climate change, extreme weather, turbulence physics, and scientific machine learning. He has received an NSF CAREER Award, ONR Young Investigator Award, and Early Career Fellowship from the National Academies Gulf Research Program.